Solutions for Improving Agricultural Productivity in the New Climate System
A.I. Crop Disease Response Solution
A.I. Crop Disease Response Solution
FAMS(Farm Artficial Intelligence Management Solution)
FAMS solutions combine artificial intelligence with ultra-high-resolution precision weather information, GIS information, and numerical modeling to improve productivity by diagnosing, predicting and responding to crop diseases in smart farms and precision agriculture.
DIGIQUAY’s automatic observation monitoring solution is a new climate system precision agricultural decision-making support solution that can provide environmental information around the farm to increase response before a disease occurs.
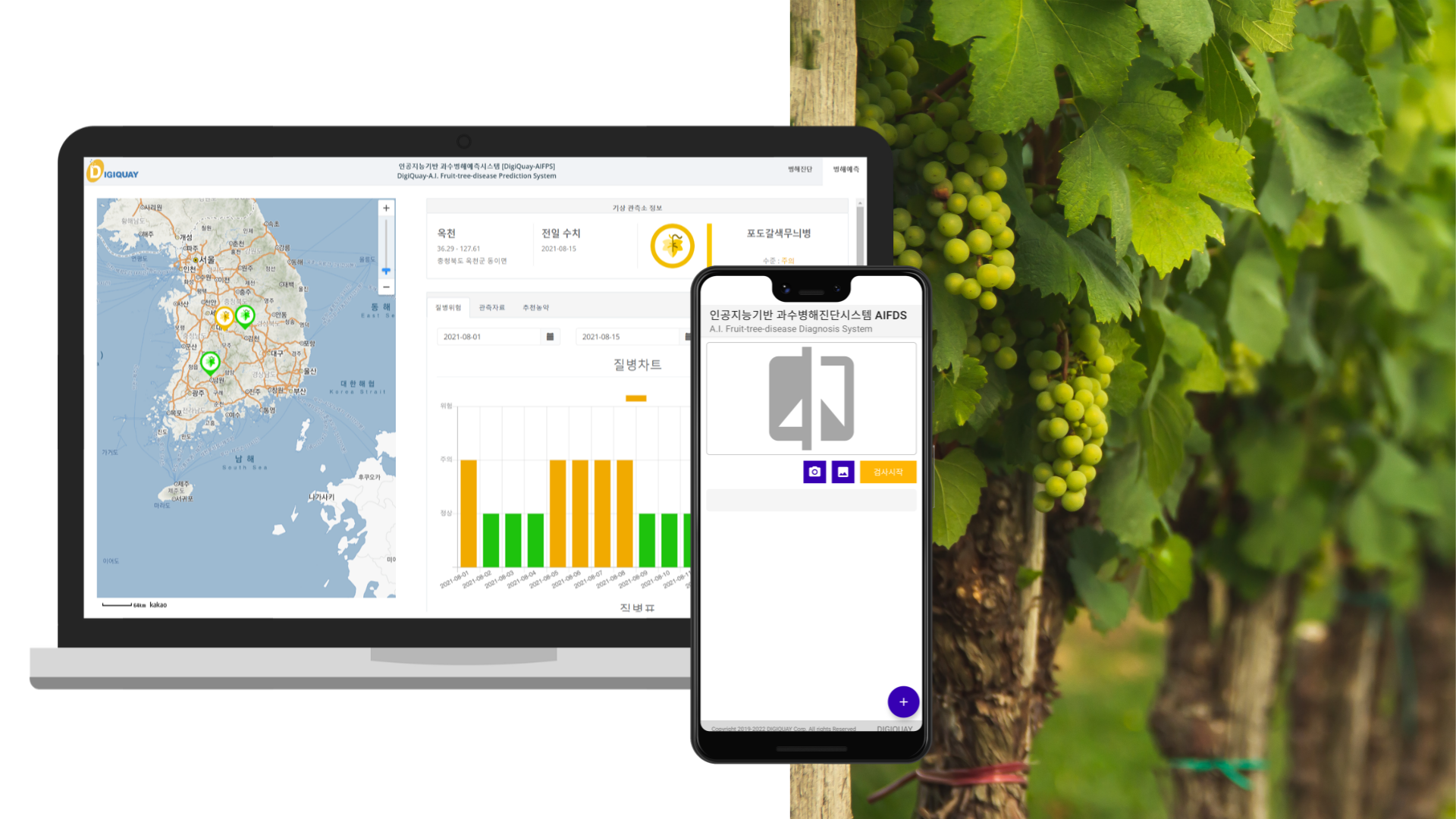
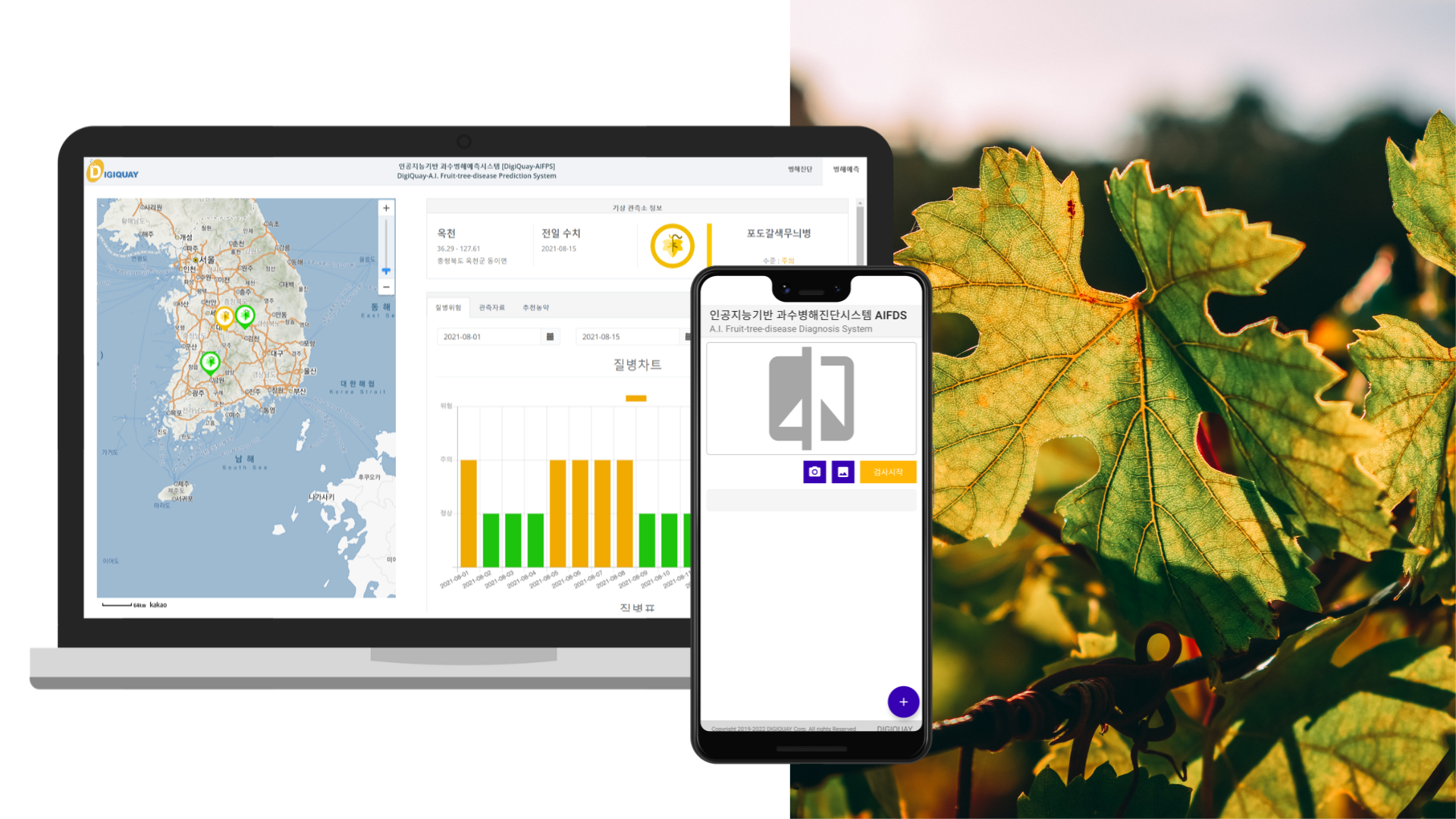
Introduction of A.I. Algorithm Machine Learning
Image data processing algorithms in the field of disease diagnosis and prediction were developed using machine learning technology to provide big data (2.6 billion data) on normal stems and leaves of fruit trees and stems and leaves where diseases occurred.
Our core technology

Data Collection

Predictive Model
/ Analysis
/ Analysis

Optimization
0
Accuracy (%)
Expectation effectiveness
Using precise agricultural weather information, it can prevent diseases, improve quality, and eliminate inefficiency through disease prediction and diagnosis, thereby reducing costs of fertilizers and pesticides needed to grow crops, reducing labor, and increasing profits from improving quality of agricultural products.
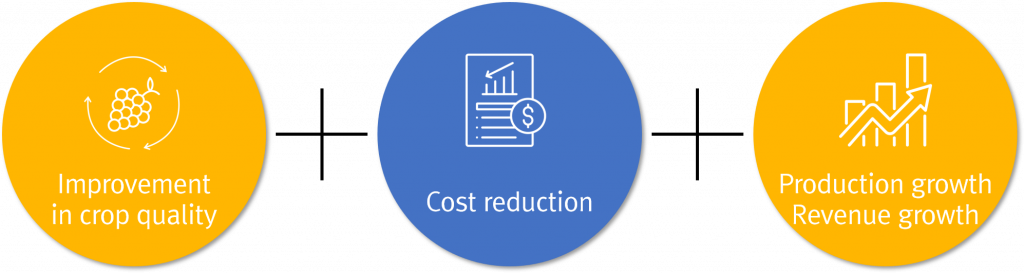
CAUCIS
Advanced, customized, ultra-high-resolution climate information services
Custom Advanced Ultra-high-resolution Climate Information Service
Custom Advanced Ultra-high-resolution Climate Information Service
Precise Agricultural Weather Prediction Information Using Its Own Technology (CAUCIS)
Traditional weather information production methods have the disadvantage of poor accuracy by using the average value of large areas. Digiki is a method of producing weather information using high-resolution topographic data based on actual weather observation data. It produces grid-type weather information at intervals of 30m by applying weights according to various topographic factors such as distance, altitude, directional plane, and ocean map.
Prediction of the occurrence of diseaseⅠ
Climate change in 2030, 2040, 2050
An example of predicting the occurrence of bluefin ulcer disease in Jeju Island with 30m ultra-high resolution
- Big Data Processing : {(A)*(B)}+{(A)*(C)*(D)}
- 23.5 * 10⁹ (23,549,762,880) data processing
- (A) Jeju Island Track and Field Grid 2,336,286 pieces
- (B) NNF analysis days: 730 days
- (C) RNI Analysis Element 24 hours
- (D) RNI Analysis Days 420 Days
Prediction of the occurrence of diseaseⅡ
Climate change in 2030, 2040, 2050
Results of predicting the occurrence of 30m ultra-high-resolution tangerine black spot disease in Jeju Island
Results of predicting the occurrence of 30m ultra-high-resolution tangerine black spot disease in Jeju Island
- Number of input data predicted probability 30m resolution
- 16.8 * 109 (16,821,259,200) data processing
- In the case of the RCP4.5 scenario, the probability of black spot disease in most coastal areas will increase by more than 60% in the 2020s, and the probability of occurrence in southern and southeastern Jeju Island is expected to be the highest

Development of optimal model technology for disease diagnosis based on convolutional neural networks
Diagnostic Accuracy of Vine Brown-Patterned Disease Image
99.36%
- Take or select a crop image
- Automatically categorize the crop as infected by entering the corresponding image into the CNN model
- Perform repetitive learning to derive the most accurate model for the lowest loss (sum of errors) value and validation data
- Loss of 0.0389 and accuracy of 0.9936 for validation data
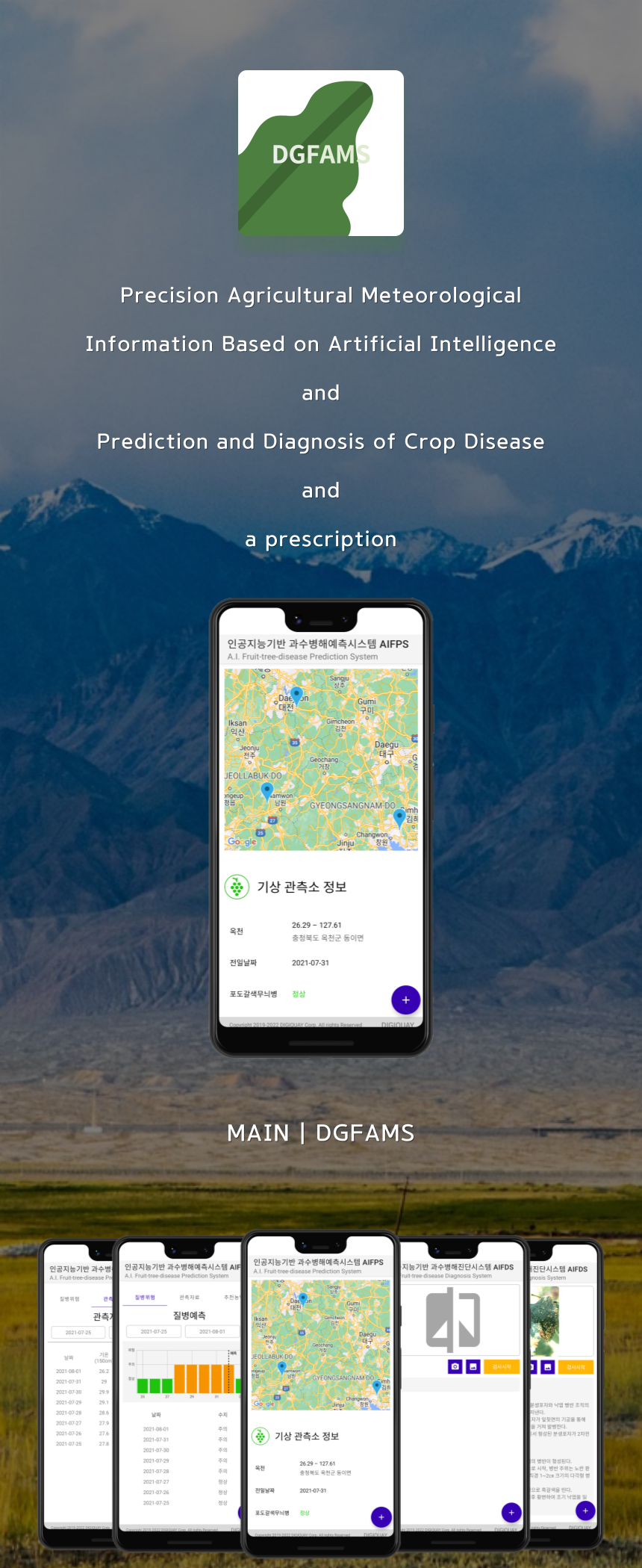

Visit Site
It is difficult to objectively judge the condition of your crops. Check out specialized solutions for disease and diagnosis.